Current Projects and Collaborations
CA21121 - European Network for the Mechanics of Matter at the Nano-Scale (MecaNano)

Our society urgently needs new materials with improved performance and durability in order to overcome its environmental crisis. Room for significant progress is available at the nano-scale, where all properties originate. Research at this length scale has strongly intensified over the past two decades, but the knowledge remains very fragmented, so that a holistic understanding of how the nanoscale mechanical behavior gives rise to the macroscopic properties of the materials is still missing.
The Action ambitions to combine the expertise and resources of European and associated researchers to overcome the different bottlenecks limiting the exploration of mechanical size effects. Synergetic gains will be achieved through a common agreement on the physical parameters to be investigated and by promoting interoperability of the research data. In addition, the experimental yield will be boosted by easing access to the latest techniques in nanomechanical testing, nanomechanical simulation and nanocharacterization to the whole community. Even more dramatic gains will be achieved by promoting the application of machine learning to nanomechanical research and favoring the development of interdisciplinary in situ techniques.
The transformative policies implemented by MecaNano will durably strengthen nanomechanical research in the European Research Area (ERA). They will foster the emergence of talented future scientific leaders, increase the number of female scientists engaging in nanoscience, as well as increase the visibility of research institutions in Inclusiveness Target Countries and allow their researchers to establish durable cooperations with their peers within the ERA.
MecaNano has been funded under Horizon Europe as a European Cooperation in Science & Technology (COST) Action for four years and is intended as a broad international cooperation aiming to advance the multiscale understanding of the mechanical behaviour of nanostructured materials.
Combining its participants’ expertise will overcome the different bottlenecks limiting the exploration of mechanical size effects. Synergetic gains will be achieved by promoting access to the latest techniques in nanomechanical testing, simulation and nanocharacterization, as well as interoperability of the research data. The long-term outcome of the Action is to unlock new possibilities for the development of complex modern materials.
Over the next four years, MecaNano will provide its members with numerous opportunities to interact and collaborate, e.g. through dedicated workshops, symposia and summer schools. It will support geographic mobility between its participants and foster the career development of its young researchers and innovators (< 40 yrs.).
The Action is opened to researchers from all countries (also beside Europe) working in the field. To become a participant, please join at least one Working Group through the following platform: https://lnkd.in/ek5pyMG3
HiDA Visiting Researcher Grant - Machine learning-enabled semantic segmentation of additive manufacturing-based structures in support of data-driven solutions

In collaboration with Prof. Yaoyao Fiona Zhao and Mutahar Safdar from McGill University we will be working on automating the process of structure information extraction in support ofsupervised learning algorithms, specifically, segmentation of structure data using Computer Vision and Deep Learning. The data can be graphic or volumetric depending on themeasurement science techniques used to evaluate the printed specimen (e.g., metallography or tomography respectively). In the proposed project, structure data in the graphic form will be segmented using Deep Learning. The data represents material systems of interest being researchedat McGill University. The proposal is also aimed at tackling new challenges introduced by the complex structures of alloys. As part of the preliminary results, the accuracy of the graphic data segmenter will be validated against ground truths from manual labeling.The project will be later aimed at researching the generalizability of such semantic segmenters, which beats the state-of-the-art.
HiDA Visiting Researcher Grant - Identification and Annotation of Defects in Atomistic Simulations
In collaboration with Ninad Bhat from the Software Innovation Institute at the Australian National University, we aim at developing automated workflows for identifying and annotating defects incrystal structure files in atomistic simulations. The project will initially focus on point defects and, upon successful proof of concept, will expand to dislocations. This project is intrinsically related to data and information science, focusing on resolving the issues of data heterogeneity and interoperability in atomistic simulations within the field of materials science. By integrating large-scale dataset generation and the workflowsdeveloped in the project, we aim to enhance the scientific utility of metadata.
Rhein-Ruhr Zentrum für wissenschaftliche Datenkompetenz (DKZ.2R)

The Rhine-Ruhr Center for Scientific Data Literacy (DKZ.2R) focuses on implementing methodological data literacy in a “holistic” manner and will actively support domain scientist in their research. The focus of DKZ.2R lies on Data Science and Machine Learning (DS/ML), High Performance Cumputing (HPC) and Research Data Management (RDM). The key domains are mathematics and computer science, life and natural sciences as well as engineering sciences.DKZ.2R consists of a number of strong project partners: Forschungszentrum Jülich GmbH, RWTH Aachen University, FH Aachen - University of Applied Sciences, Universität Bonn, Fraunhofer Institute for Intelligent Analysis and Information Systems (IAIS), Hochschule Bonn-Rhein-Sieg - University of Applied Sciences, University of Cologne, Ruhr-University Bochum, and University Duisburg-Essen, and benefits from a close connection to the Helmholtz School for Data Science in Life, Earth and Energy (HDS-LEE), the German National Research Data Infrastructure (NFDI), the LAMARR Institute for Machine Learning and Artificial Intelligence, the state initiative for research data management (fdm.nrw), and many more.
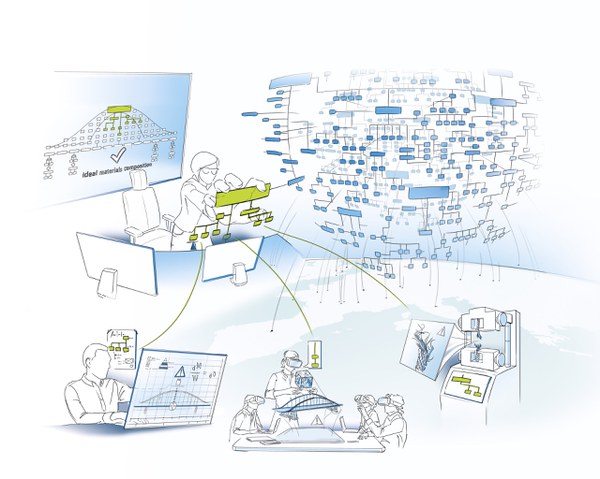
NFDI-MatWerk
Since industrialization, our social and economic progress depends on the mastery of materials. From the very beginning, materials science and materials engineering – in German: Materialwissenschaften und Werkstofftechnik = MatWerk – have been key technologies of their time. Due to their versatility, these two interdisciplinary fields hold a wealth of innovative solutions to meet societal challenges in the future fields of energy, mobility, environment, etc., thus making a significant contribution to the sustainable use of our finite resources. One challenge here is the many structural scales and thus the various experimental and numerical methods. In turn, materials’ mechanical and functional properties are determined by their microstructure and thus also by likely changes due to their process and load histories. The development of a database infrastructure is a community-driven process. To this end, NFDI-MatWerk aims to seamlessly integrate decentralized data and metadata, experimental and numerical workflows, and a materials ontology to maximize interoperability and reproducibility of research data processing. To this end, data use profiles of Participant Projects from different sub-disciplines are analyzed to identify the most relevant scientific scenarios within MatWerk. The resulting Infrastructure Use Cases help in the continuous community-driven development and review of the infrastructure.
Control of the microstructure of thin multilayer systems by ultrashort pulsed laser irradiation - process understanding by complementary in situ and ex situ characterizations and multiscale simulations

The aim of this DFG research grant is to contribute to the understanding of laser-induced changes in the microstructure of thin metallic films and to the description of the effect of microstructure on the materials characteristics, which are relevant for the laser processes, e.g., absorption of the laser beam, electron-phonon coupling, heat transfer, etc. This aim should be achieved by combining in situ (ultrafast ellipsometry and reflectometry during the laser irradiation) and ex situ experiments (scanning and transmission electron microscopy, X-ray and electron spectroscopy) with simulations using mesoscopic (hydrodynamics) and microscopic (molecular dynamics) approaches. The evaluation of the electron micrographs will be supported by a multimodal analysis based on deep learning. The information obtained from the molecular dynamic simulations will complement the ex situ microstructure studies by providing, e.g., the atomic positions for the in situ microstructure analyses during the laser irradiation.The materials proposed for this study are single layers (Cr, Mo, Ti, Fe) and bilayers (Au/Cr, Mo/Ti, Au/Fe) consisting of unary metallic phases with different melting points, different sequences of high-temperature and high-pressure phases, and with different mutual solubilities and diffusivities in the respective binary system. Experimentally observed phase transitions and concentration profiles will be used as “sensors” for the temperatures and pressures induced by the laser irradiation. The effect of the microstructure on the laser process will be studied in samples having different grain size and preferred orientation of crystallites in the original state. ng the laser irradiation.
Helmholtz Imaging Project - Deep-learning assisted fast in situ 4D electron microscope imaging (FAST-EMI)
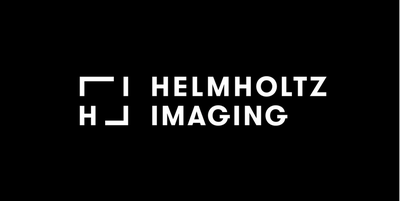
Together with Prof. Dr. Christoph Kirchlechner and Dr. Subin Lee from Karlsruhe Institute of Technology, our vision is to create tailored imaging approaches that unlock the full potential of in situ 4D-STEM-in-SEM. Therefore, we are developing a model to couple a novel 4D-STEM-in-SEM measurement method with deep learning algorithms in order to improve the temporal resolution of the measurements and to significantly shorten the measurement duration.

Helmholtz Metadata Collaboration
The HMC Hub Information works towards optimized metadata that allows researchers to connect in cross- and multidisciplinary collaborations. We help to bridge the gap between discipline specific problems by endorsing, adjusting and developing cross-disciplinary solutions for the creation, curation and management of scientific metadata.
The Working Package FAIR Data Commons: Technologies and Processes facilitates compliance with the FAIR principles for research data coming from all scientific fields covered by the Helmholtz Association and beyond. It will foster uniform access to metadata by providing standardized interfaces, which are in line with recommendations and standards adopted by global research data initiatives, e.g. the Research Data Alliance (RDA). These interfaces will be accomplished by easy-to-use tools, generally applicable processes, and best practices in order to support scientists in their daily work. In addition, training and consultancy services will be offered in order to make the transition to FAIR research data even easier.

Electron Microscopy Data Science Lab
This interdisciplinary simulation and data science lab, co-hosted by the Ernst Ruska-Centre for Microscopy and Spectroscopy with Electrons (ER-C) and lead by the IAS-9, is initiated to create added value by combining competencies and know-how in data science, computational materials science as well as research data management, all with a focus on the field of electron microscopy.
Helmholtz School for Data Science in Life, Earth, and Energy

HDS-LEE is an international, English-speaking graduate school and a cooperation between RWTH Aachen University, the University of Cologne, the German Aerospace Center (DLR), the Max-Planck-Institut für Eisenforschung, and Forschungszentrum Jülich. HDS-LEE is part of the newly founded JARA Center for Simulation and Data Sciences (JARA-CSD), which will be created as a unique, internationally visible competence center for computer- and data-infrastructures, user support as well as methodological and disciplinary research in the fields of simulation, data analysis and HPC technologies.